In the rapidly changing finance sector, the potential of Artificial Intelligence (AI) and predictive analytics to revolutionise planning processes has been a hot topic among professionals. Despite widespread recognition of their transformative power, a perplexing paradox remains firmly in place: while the value of AI is almost universally acknowledged, its adoption in algorithmic planning is lagging. This article aims to dissect this paradox, illuminating the underlying challenges and charting a path forward for finance teams. Extensive research and reports from leading consulting and technology firms have revealed two distinct, yet contrasting, trends:
-
- An overwhelming 93% of these teams believe in the significant value that AI can bring to their processes.
- Less than 20% of finance teams are either using or experimenting with predictive analytics in their operational planning.
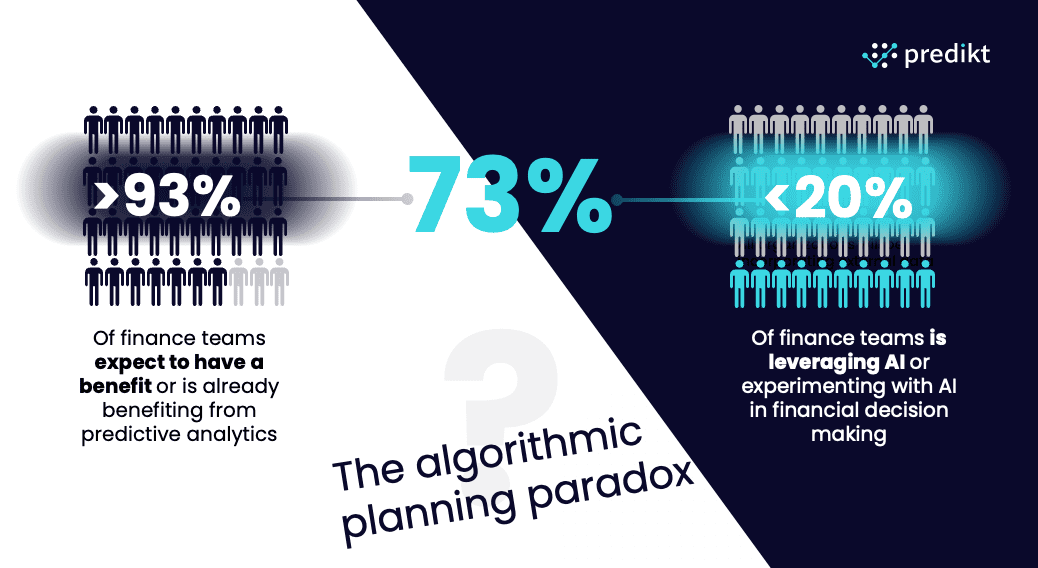
This discrepancy – 73% of the finance leaders believe in AI, but not using it underscores a persistent paradox, one that has been the subject of discussion and analysis by powerhouses like Deloitte , PwC , EY , KPMG , Accenture , Bain & Company , McKinsey & Company , and Boston Consulting Group (BCG) for years. Despite numerous advisory services and proof-of-concept projects, a broad-based shift toward algorithmic planning remains elusive.
Why is there such a gap between the recognised value of AI and its practical implementation within finance teams?
At Predikt, we believe that the answer lies in the absence of technology that fully meets the needs of these teams. Several critical areas require attention before finance teams can embrace advanced analytics on a large scale.
Comprehensive Data Utilisation
One of the primary shortcomings of current solutions is their inability to fully harness the spectrum of available data for making informed decisions. This results in notable blind spots. Decisions in the financial realm have traditionally been based on historical financial data, with more progressive organisations also incorporating internal business and company-specific drivers like full-time equivalents (FTEs), sales volumes, etc., based on straightforward relationships. However, the integration of external micro- and macroeconomic indicators into forecasting models remains rare. Such indicators could significantly enhance decision-making, especially in times of economic volatility. The question then becomes: How can finance teams make truly data-driven decisions without considering all readily accessible internal and external data?
Efficiency and Time-to-Value
The journey to implement advanced analytics is fraught with challenges, not least of which is the time it takes to see tangible value from these models. From the moment data is collected (which is typically a first challenge), it must undergo various preprocessing, cleansing, and augmentation steps before it can be deemed ready for use in AI models. The field of machine learning and time-series forecasting is vast, with thousands of algorithms each capable of being calibrated in countless ways. The complexity involved in constructing a truly effective and stable model is significant, and finance teams often lack the resources to employ a team of AI engineers. Furthermore, even when an algorithmic model does begin to show value, the ongoing expenses of maintenance and updates can be prohibitively high and sponsors stop the initiative and take the sunk cost.
Beyond Accuracy
A singular focus on model accuracy overlooks other critical dimensions of performance evaluation. It’s relatively straightforward to tweak a model to achieve high accuracy, even up to 99%, by continuously adding data until it perfectly fits the historical data. However, such an approach risks overfitting, where the model is so finely tuned to past data that it fails to perform when confronted with new information. There are numerous other metrics that should be monitored to ensure a model’s robustness and reliability over time. The fixation on accuracy alone can lead to misplaced confidence in a model’s predictive power, particularly when it fails to adapt to fresh data.
The Causation Key
A significant reliance on correlation tests to establish relationships between variables often overshadows the vital distinction between correlation and causation in current predictive analytics solutions. While correlation can indicate a relationship between two variables, causation goes a step further by asserting that one variable directly influences the changes in another. This distinction is critical, as relying solely on correlation can lead models to draw connections that, while statistically significant, lack logical underpinnings or causal links. Such models might identify bizarre indicators as predictors, devoid of any logical business rationale for why one variable would affect another. This not only undermines the credibility of the models but also contributes to a hesitancy within finance teams to trust and adopt AI-driven solutions.
Reflecting Business Knowledge
Many of the current predictive models fail to account for the nuanced interdependencies that characterise a business’ financial ecosystem. For instance, when analysing revenue increases due to an uptick in an external indicator, should costs be expected to remain stable? Existing models tend to treat each target variable (such as revenue, costs, volumes) in isolation, resulting in predictions that fail to reflect the interconnected reality of business operations. This approach overlooks direct, deterministic relationships between variables, simplifying complex interactions into basic functions without recognizing the inherent linkages between them, such as revenue being the product of price and volume. This oversimplification can make it challenging to reconcile differences between AI-predicted outcomes and those derived from human planning, complicating the understanding of whether discrepancies are due to volume underestimations or inaccurate price predictions.
Integrating Human Insight
The prevailing mindset often frames the relationship between AI forecasts and human judgement as an either/or scenario, failing to capitalise on the potential for synergistic integration. Most AI forecasting models are perceived as black boxes, making it difficult to compare the reasoning behind human-generated forecasts with that of the machine. For predictive analytics to truly deliver its full potential, it’s imperative to develop models that not only replicate an unbiased human thought process but also enhance it by seamlessly incorporating machine-generated insights. This approach requires models to be designed in a way that augments planners’ capabilities, facilitating a harmonious blend of human intuition and algorithmic precision.
Conclusion
The paradox of algorithmic planning in finance underscores a critical juncture in the adoption of AI and predictive analytics. Bridging the gap between current technological capabilities and the requirements of finance teams is essential for unlocking the full potential of these advanced tools. By addressing the key challenges and focusing on a more integrated, holistic approach, the finance sector can navigate the complexities of the digital age and harness the power of algorithmic planning to drive strategic decision-making and growth.